A comprehensive study on the prognostic value of pyroptosis-associated genes in hepatocellular carcinoma
Abstract
Aim: Hepatocellular carcinoma (HCC) is a prevalent malignancy globally, and pyroptosis, an inflammatory form of programmed cell death, is closely associated with tumor progression. The aim of this study is to explore the functional role of pyroptosis in HCC progression.
Methods: RNA sequencing and clinical data of HCC patients from TCGA and GEO databases were analyzed. We examined the expression patterns of 49 pyroptosis-related genes (PRGs) in HCC. Univariate Cox regression and consensus clustering divided TCGA-HCC patients into two subtypes, C1 and C2. A prognostic model was developed using LASSO Cox regression based on significant PRGs, and predictive value was assessed through nomogram and decision curve analysis (DCA). GSEA and immune infiltration analysis were conducted to evaluate immune status. Additionally, Networkanalyst tools were used to predict regulating networks of PRGs, and gene expression was validated using qRT-PCR.
Results: Subtype C2 showed higher grades (III-IV), immune scores, genetic mutations, and increased immune factor expression. A prognostic model based on four prognostically relevant PRGs stratified HCC patients into high- and low-risk groups. The low-risk group had better survival. The risk score was an independent prognostic factor with strong predictive ability. Immune status differed between the two risk groups. Regulatory networks between PRGs, transcription factors (TFs), miRNAs, and chemicals were identified. qRT-PCR confirmed higher expression of PRGs in paracancerous tissues compared to carcinoma.
Conclusion: A prognostic model based on four PRGs offers significant implications for prognosis assessment and could inform HCC treatment strategies.
Keywords
INTRODUCTION
Primary liver cancer is a highly diverse and lethal malignancy with significant histological and biological variations and is the fourth leading cause of cancer-related mortality worldwide. Hepatocellular carcinoma (HCC) represents the predominant subtype, encompassing approximately 80% of all primary liver cancer instances[1,2]. HCC originates from hepatocytes, forming dense, solid, and trabecular structures. Key risk factors include liver cirrhosis, viral hepatitis, alcohol, diabetes, non-alcoholic fatty liver disease, metabolic syndrome, and environmental toxins. Its progression involves chronic inflammation due to liver damage, leading to hepatocyte death, regeneration, and fibrosis[3,4]. Mechanistic studies on HCC reveal the roles of tumor suppressor genes (e.g., p53), oncogenes (e.g., K-ras), activated signaling pathways (PI3K, MAPK, JAK/STAT, NF-κB, Wnt/β-catenin), and exosomes carrying pro-tumorigenic molecules in its development[5]. Consequently, understanding the mechanisms of HCC onset and progression, along with identifying prognostic markers, is crucial for effective prevention, early detection, and treatment.
Cell death is critical for discovering antitumor drugs. Pyroptosis, a novel form of programmed cell death, involves Gasdermin-mediated pore formation, membrane rupture, cellular lysis, and the release of pro-inflammatory substances[6,7]. Pyroptosis occurs through two pathways: the caspase-1-mediated canonical inflammasome and the caspase-4/5/11-mediated noncanonical inflammasome. It serves dual functions: protecting organs from infection or danger, and causing pathological inflammation when excessively activated[8]. Studies show pyroptosis plays a key role in tumorigenesis and progression. It can both inhibit tumor formation and alter the tumor microenvironment, promoting cancer growth[9]. In HCC, the numbers of immune cells and the levels of immune-stimulating molecules are increased by pyroptosis in liver cancer cells[10]. Additionally, it has been reported that pyroptosis is inhibited in HCC tissues and cells[11]. Furthermore, the NLR pyrin domain containing 3 (NLRP3) inflammasomes, associated with caspase 1-dependent pyroptosis, are significantly downregulated in HCC cells[12]. However, the specific functions of pyroptosis in the HCC development and prognosis remain unclear.
This study explores the role of pyroptosis-related genes (PRGs) in predicting HCC prognosis and guiding treatment. We analyzed PRG expression patterns in HCC and normal tissues, identified two subtypes, and developed a prognostic risk model using LASSO Cox analysis. We also examined the model's clinical value, the link between pyroptosis and tumor immune infiltration, and constructed regulatory networks for prognostic PRGs. This study offers new targets and strategies for HCC prognosis and treatment.
MATERIALS AND METHODS
Data acquisition and processing
We obtained the gene expression (fragments per kilobase million, FPKM) data, genomic mutation data, and the corresponding clinical features of 414 HCC samples from the UCSC Xena database
Identification of differentially expressed PRGs and functional annotation
The “limma” package was used to identify differentially expressed genes (DEGs) from PRGs between HCC and normal tissues. DEGs were visualized by the boxplot with an adjusted P-value < 0.05. DEGs were then performed by functional enrichment analyses using the Metascape website
Analysis of molecular subtypes
The cluster analysis of HCC patients in the TCGA cohort was conducted using the “ConsensusClusterPlus” package in R software. Then, the differential expression pattern of DEGs between clusters was identified by the R package “limma” with FDR < 0.05 and |log2FC| > 1. Heatmaps of differential DEGs between clusters were drawn using the R-package, pheatmap. The immune infiltration scores for TCGA-HCC patients, including stromal, immune, and ESTIMATE scores, were calculated using the “ESTIMATE” package in R. The violin plots drawn by the “ggplot2” package exhibited the differences in immune infiltration between clusters. Moreover, we predicted a semi-inhibitory concentration (IC50) value to explore differences in the therapeutic effects of drugs in HCC patients using the “pRRophetic” package based on the GDSC (Genomics of Drug Sensitivity in Cancer) database. Subsequently, the TISIDB platform was utilized to investigate immunosuppressive factors, immunostimulatory factors, chemokines, and receptors with significant differences between subtypes. Functional enrichment analysis using Gene Ontology (GO) and the Kyoto Encyclopedia of Genes and Genomes (KEGG) was carried out with the clusterProfiler package in R, and P-values < 0.05 were considered statistically significant.
Construction and validation of the pyroptosis-related gene prognostic model
First, the PRGs were subjected to univariate Cox regression analysis to screen those correlated with HCC prognosis, and the cutoff P-value was set at 0.05. To obtain an optimal prognostic model, multivariate Cox regression analysis was applied to optimize the prognostic PRGs using the Least Absolute Shrinkage and Selection Operator (LASSO) regression model. The “stepAIC” algorithm in the “MASS” R package was also employed for stepwise regression performance to identify the most contributable PRGS to the model. Based on the variables gained from LASSO and the regression coefficients, the prognostic model was defined as: risk score = sum of coefficients × PRG expression. We next divided the HCC patients into high- and low-risk groups according to the median risk score. The survival analysis between the two risk groups was performed using the “survival” and “survminer” R packages. The receiver operation characteristic (ROC) curve analysis of 1, 3, and 5 years was used to predict the efficiency of the prognostic model through the “survivalROC” R package.
Development of a predictive nomogram
We first explored the association between the clinical characteristics (Age, Gender, Grade, Race, Stage, BMI, Prior malignancy) and the risk score calculated from the prognostic model through univariate and multivariate Cox regression analyses. Then, a nomogram was constructed to predict the overall survival of HCC patients over 1, 3, and 5 years using the “rms” and “survivalROC” packages. Subsequently, ROC and calibration curves were plotted to test the nomogram's validity and reliability with “survminer” and “timeROC” packages. The “ggDCA” package was utilized for decision curve analysis (DCA) to validate the clinical value of the predictive nomogram.
Gene sets enrichment analysis
Gene set enrichment analysis (GSEA) was performed to identify the biological pathways that were significantly changed between the high-risk and low-risk groups using the “clusterProfiler” R package. The gene set was obtained from “c2.cp.kegg.v7.4.symbols.gmt” of the Molecular Signatures Database (MSigDB, https://www.gsea-msigdb.org/gsea/index.jsp). The parameters utilized in the GSEA for enrichment analysis are as follows: the number of permutations was set to 1,000, and each gene set was required to contain a minimum of 10 genes and a maximum of 500 genes. The p-value correction method employed was the Benjamini-Hochberg method (BH). A significance threshold was set at P < 0.05 and FDR < 0.05, indicating significant enrichment.
Immune infiltration and multi-omics network analysis
The Cell-type Identification by Estimating Relative Subsets of RNA Transcripts (CIBERSORT) algorithm was implemented to quantify the abundance of 22 infiltrating immune cells in HCC patients of two subgroups. The mRNA-TF (Transcription Factor), mRNA-miRNA, and mRNA-chemical interactions were predicted with Networkanalyst online tools, which specialized in transcriptome profiling, network analysis, and meta-analysis for gene expression data. The mRNA-TF network was established using the JASPAR database. The mRNA of the associated miRNA was analyzed using the TarBase database (V8.0). The protein-chemical interactions are presented by the comparative toxicogenomics database (CTD).
Real-time PCR for mRNA quantitation
Total RNA from HCC tissues was isolated using Trizol reagent (Invitrogen, United States) according to the manufacturer’s protocol. Reverse transcription of the extracted RNA was performed by using First-Strand cDNA Synthesis Super Mix for qPCR with one-step gDNA remover (AmeriDx, United States). The SYBR Green-based real-time qPCR was carried out using the indicated primer pairs for each target gene
Statistical analysis
All statistical analyses were accomplished with R (version 4.2.1). The Pearson chi-square test was used to compare the categorical variables. The Spearman’s and distance correlation analyses were performed to calculate the correlation coefficients. The significance of differences in survival curves was identified by the two-sided log-rank tests. Statistical significance was set at P < 0.05.
RESULTS
Landscape of genetic variations of PRGs in HCC
First of all, we explored the expression of the 49 PRGs between the normal liver and HCC samples using the TCGA cohort. A total of 37 pyroptosis-related DEGs were identified, all showing statistically significant differences in expression (all P-values < 0.05). Among them, the expression of 27 genes (APIP, CASP3, CASP4, CASP6, CASP8, CASP9, CNOT6, CSDE1, DHX9, DPP9, DRD4, GPX4, GSDMB, GSDMC, GSDMD, GSDME, NLRP1, NOD1, NOD2, PELO, PJVK, PLCG1, PRKACA, PYCARD, SCAF11, TIRAP and TREM2) were upregulated, while 10 genes (AIM2, ELANE, IL1β, IL6, MEFV, NLRC4, NLRP3, NLRP6, NLRP7 and NLRP9) were downregulated in HCC tissues [Figure 1A and B]. Subsequently, functional enrichment analysis using the Metascape website showed 37 DEGs were mainly clustered in pyroptosis, inflammasome complex, regulation of defense response, response to lipopolysaccharide, and defense response to Gram-positive bacterium [Figure 1C]. At the genetic level, 74 of the 364 samples (20.33%) represent pyroptosis-related alterations, and missense mutation was identified as the most common variant classification. DHX9, NLRP3, and NLRP7 were the top 3 genes with the highest frequency of mutations [Figure 1D]. Correlations were performed between each DEG, and statistically significant correlations were identified from the correlation matrix heat map. GPX4 was discovered to be negatively correlated with DHX9 and SCAF11. PYCARD had a significant positive correlation with TREM2 and CASP4. In addition, a positive correlation between AIM2 expression and NLRC4 and NLRP3 expression was found [Figure 1E]. Next, we constructed the protein-protein interaction (PPI) network using STRING to study the potential biological functions of DEGs. The network indicated that GSDMD, CASP4, and PYCARD strongly interact with other DEGs and are of high importance [Figure 1F].
Figure 1. Genetic alterations and expression patterns of PRGs in HCC. (A and B) Boxplots showing the FPKM expression levels of PRGs in normal versus HCC samples. Tumor, yellow; Normal, blue. (C) Metascape visualization of the networks of the top 20 clusters. Each node represents an enriched term, with node color indicating the cluster ID. Nodes within the same cluster tend to be spatially proximate. Node size reflects the number of input genes associated with the term, and thicker edges indicate greater similarity between terms. (D) Mutation frequency and classification of 37 differentially expressed pyroptosis-related DEGs in 364 HCC patients. (E) Heatmap of the correlation matrix showing pairwise correlations among the 37 DEG. (F) PPI network of the 37 DEGs generated using the STRING database. *P < 0.05, **P < 0.01, ***P < 0.001, ****P < 0.0001.
Verification of the expression of PRGs in carcinoma, paracancerous tissue and paired normal tissue in liver
We collected tissue samples from three HCC patients to validate the expression of certain important PRGs. These three patients were in stage II or III and had not received chemotherapy [Figure 2A]. The expression of 9 PRGs, including APIP, CASP3, CASP4, CASP6, CASP8, NOD1, NOD2, PELO, CASP9, DPP9 and NLRP1, were verified through quantitative reverse transcription polymerase chain reaction (qRT-PCR). The results determined that the expression of 9 PRGs had an increasing trend in paracancerous tissues compared with normal liver tissues. In liver carcinoma, the relative expression levels of CASP6, NOD2, and CASP9 were significantly lower than in paracancerous or normal liver tissue [Figure 2B]. When the mRNA expression was compared in paired samples of liver carcinoma to paracancerous tissue and paired normal tissue, the mRNA ratios of 9 PRGs upregulated between carcinoma/normal tissue and paracancerous tissue/ normal tissue. We also observed that the mRNA ratios of CASP3, CASP6, DPP9, and NLRP1 in carcinoma/paracancerous tissue were remarkably lower than in paracancerous tissue/normal tissue [Figure 2C]. The results suggested that PRGs were more highly expressed in paracancerous tissues than in carcinoma tissues of HCC, which might play an important role in the development and progression of HCC.
Figure 2. Expression of PRGs in HCC, paracancerous tissue and paired normal liver tissue. (A) Clinical features of three HCC patients for validating PRG expression. (B) qRT-PCR of HCC, paracancerous tissue, and paired normal liver tissue. mRNA quantification of APIP, CASP3, CASP4, CASP6, CASP8, NOD1, NOD2, PELO, CASP9, DPP9, and NLRP1 was normalized to GAPDH. (C) mRNA ratios between HCC and paracancerous tissue and paired normal liver tissue within the same patients. Each group consists of three independent replicates. Data are presented as mean ± SEM.*P < 0.05; **P < 0.01.
Identification of HCC subtypes and their prognostic value
To investigate the relationship between PRGs and HCC subtypes, the consensus clustering method was used to explore HCC subtypes depending on the expression of 49 PRGs. According to the cumulative distribution function (CDF) value, we determined the optimum k value was 2 and divided the HCC samples into two subtypes, C1 and C2 [Supplementary Figure 1].
Between the TCGA-HCC patients from the subtypes C1 and C2, the expression of 485 genes differed, and 304 of them showed a tendency to be upregulated, while 181 genes were downregulated in subtype C2 [Figure 3A]. Then, functional enrichment analyses of 485 DEGs were performed to figure out the differences in biological functions and pathways between the two subtypes. Gene ontology (GO) enrichment analysis of the biological process category revealed that DEGs were related to leukocyte migration, the biosynthetic processes of carboxylic and organic acids, and small molecule catabolic processes, showing differential expression between the two subtypes [Supplementary Figure 2A]. In the molecular function category, DEGs were predominantly enriched in monooxygenase activity, endopeptidase inhibitor activity, and iron ion binding [Supplementary Figure 2B]. Cellular component enrichment analysis showed significant enrichment in the collagen-containing extracellular matrix, MHC class II protein complex, and cytoplasmic vesicle lumen [Supplementary Figure 2C]. Furthermore, KEGG pathway analysis indicated that the DEGs were primarily involved in complement and coagulation cascades, Staphylococcus aureus infection, rheumatoid arthritis, and the metabolism of xenobiotics by cytochrome P450 [Supplementary Figure 2D].
Figure 3. TCGA- HCC molecular subtypes based on PRGs and their characteristics. (A) Volcano plot of DEGs between the two subtypes in the TCGA cohort. The yellow dots represent upregulated DEGs, the blue dots indicate downregulated DEGs, and the grey dots stand for undifferentiated expression genes. (B) Heatmap of PRGs between the subtype C1 and subtype C2. (C) The ESTIMATE score, immune score, and stromal score demonstrate the distinct immune statuses between the two subtypes. (D) Boxplots showing the drug sensitivity of Axitinib, Bosutinib, Erlotinib, and Gefitinib between the two subtypes. (E and F) Oncoplot displaying the somatic landscape of HCC with subtypes C1 (E) and C2 (F). 15 genes were sorted with the highest mutation. ****P < 0.0001.
We also discovered that the expression patterns of PRGs were significantly different between the two subtypes [Figure 3B]. Next, the distribution of clinical characteristics including Age, Gender, Grade, Race, Stage, BMI, and Prior Malignancy between subtypes is displayed in Supplementary Table 3. Interestingly, 42.9% of patients in subtype C2 were in stages III-IV, much higher than the 31.2% observed in subtype C1, indicating the faster-growing tumors in subtype C2. To explore the correlation between the two subtypes and tumor immune status, the ESTIMATE algorithm was implemented to calculate the ESTIMATE score, immune score, and stromal score. The results demonstrated the subtype C2 had higher scores [Figure 3C]. Furthermore, the prophetic algorithm was applied to assess the chemosensitivity of Axitinib, Bosutinib, Erlotinib, and Gefitinib between subtypes according to the GDSC database. Notably, patients in subtype C1 may benefit more from Bosutinib and Gefitinib treatment, while Axitinib and Erlotinib may provide a more favorable treatment for patients in subtype C2 [Figure 3D]. To evaluate the distribution differences of somatic mutation between subtypes C1 and C2 in the TCGA-HCC cohort, we identified the first 15 most frequently mutated genes in HCC patients and compared the mutation burden. The subtype C1 presented a higher mutation burden than the subtype C2 [Figure 3E and F].
Subsequently, the expression levels of immune factors, including receptors, immune inhibitors, immunostimulants, and chemokines, were analyzed. Most receptors, immune inhibitors, and immunostimulants were highly expressed in the subtype C2 [Figure 4A-C]. For chemokines, CCL14, CCL15, and CCL16 showed remarkably increased expression in subtype C1, while others showed elevated expression in subtype C2 [Figure 4D and E]. These results suggested that HCC in subtype C2 might be more closely correlated with immunity than in subtype C1.
Construction and validation of the effectiveness of the prognostic risk model
To develop a prognostic risk model, Univariate Cox regression analysis was performed for primary screening of 49 PRGs, identifying 17 DEGs (APIP, MEFV, TREM2, GSDMC, NLRP6, GZMA, DHX9, GSDME, CASP8, NLRC4, CSDE1, PELO, CNOT6, CASP5, NOD1, NOD2, SCAF11) related to prognosis for further analysis (with P < 0.05). Next, a multivariate Cox regression analysis was performed to construct the prognostic risk model, which was then optimized using the stepAIC algorithm. The risk score was calculated according to the expression level and regression coefficient of four genes (TREM2, GZMA, CNOT6, and NOD2): risk score = (TREM2 × 0.2349949) + (GZMA × -0.3606756) + (CNOT6 × 0.5372685) + (NOD2 × 0.4743908). Based on the median score calculated by the risk score formula, patients in the TCGA training cohort and validation cohorts (GSE14520 and GSE116174) were stratified into high- and low-risk groups. Kaplan-Meier curves were applied for survival analysis and revealed that HCC patients with high-risk scores had a worse overall survival probability than those with low-risk scores [Figure 5A-C]. To evaluate the sensitivity and specificity of the prognostic model, time-dependent receiver operating characteristic (ROC) analysis was used. The area under the ROC curve (AUC) at 1, 3, and 5 years was 0.756, 0.697, and 0.659, respectively, on the TCGA samples [Figure 5D]. The AUCs were 0.659 for 1 year, 0.711 for 3 years, and 0.61 for 5-year survival on GSE14520 samples [Figure 5E]. HCC patients in the GSE116174 dataset were accompanied by AUCs of 0.676, 0.648, and 0.737 in the 1-year, 3-year, and 5-year ROC curves, respectively [Figure 5F]. These results suggested the prognostic model had a good predictive ability. In addition, the risk score and survival time scatter plots were drawn to study the different trends of survival status between two risk groups on the TCGA, GSE14520, and GSE116174 datasets. Patients in the high-risk group showed a higher proportion of deaths and a shorter survival time than those in the low-risk group
Figure 5. Development and validation of the prognostic risk model. (A-C) The KM analysis of the overall survival in the high-risk and low-risk groups on the TCGA (A), GSE14520 (B), and GSE116174 (C) datasets. (D-F) ROC curves of the TCGA (D), GSE14520 (E), and GSE116174 (F) datasets. (G-I) The relationship between survival status and risk score for each patient on the TCGA (G), GSE14520 (H), and GSE116174 (I) datasets. Dots on the left side of the dotted line represent the low-risk population, while those on the right side stand for the high-risk population. KM: Kaplan-Meier; ROC: receiver operating characteristic.
Establishment of a prognostic nomogram and gene set enrichment analysis
We utilized univariate and multivariable Cox regression analyses to determine whether the risk score and clinical features could be independent prognostic factors. We discovered that the risk score and stages III&IV were independent factors, with the greater the risk score or higher the stage, the poorer the prognosis [Figure 6A and B]. Subsequently, a novel prognostic nomogram was developed based on the risk scores and stage, which could determine the 1-, 3-, and 5-year overall survival rates [Figure 6C]. Kaplan-Meier (KM) analysis indicated that scoring by the nomogram discriminated the risk groups effectively [Figure 6D]. The AUCs of the nomogram at 1, 3, and 5 years were 0.773, 0.743, and 0.706, respectively, suggesting the good predictive power of the nomogram model
Figure 6. Construction of nomogram prognostic model. (A and B) The univariate (A) and multivariate (B) Cox regression analyses evaluated the independent prognostic values. (C) The nomogram integrated the risk score to predict the overall survival in the HCC patients. (D) Overall survival analysis for HCC patients stratified by the prognostic nomogram using KM curves. (E) The time-dependent ROC curves for the nomogram. (F) The DCA curve of the prognostic nomogram. HCC: Hepatocellular carcinoma; KM: Kaplan-Meier; ROC: receiver operating characteristic; DCA: decision curves analysis.
To identify the potential functional differences between the high-risk and low-risk groups, GSEA analysis was carried out using the TCGA dataset. The gene sets are mainly enriched in the PI3K-Akt signaling pathway, Human papillomavirus infection, Pathways in cancer, Endocytosis, Shigellosis, and Salmonella infection [Figure 7].
Figure 7. Enrichment plots from gene set enrichment analysis. (A) The top 20 gene sets significantly enriched. (B-G) Representative enrichment plots generated by GSEA. PI3K-Akt signaling pathway (B); Human papillomavirus infection(C); Pathways in cancer (D); Endocytosis (E); Shigellosis (F); Salmonella infection (G).
Immune cell infiltration and analysis of regulating networks
To further investigate whether prognostic-related PRGs affect immune cells, the correlations between immune cells and genes in the risk model were plotted in the heatmap. M0 Macrophages were negatively related to GZMA, while resting dendritic cells were positively correlated with TREM2. Additionally,
Figure 8. Immune cell infiltration landscape. (A) Heatmap showing the correlations between immune cells and prognostic PRGs. (B) Cellular interaction network of the 22 immune cell types. (C) Heatmap displaying differences in immune cell profiles between high- and low-risk groups. (D) Boxplots showing the composition of immune cells in the high- and low-risk groups. (E) Differential infiltration levels of 22 immune cell types between the two subgroups. *P < 0.05, **P < 0.01, ****P < 0.0001.
The construction of regulated networks involving the mRNAs of 4 prognostic PRGs (TREM2, GZMA, CNOT6, and NOD2), along with TFs (transcription factors), miRNAs, and chemicals, provided valuable insights into the regulatory role of pyroptosis in HCC. We first constructed the mRNA-TF regulatory network and identified 23 TFs [Figure 9A]. In the mRNA-chemical regulatory network, 35 chemicals were identified [Figure 9B]. The mRNA-miRNA regulatory network revealed 136 miRNAs [Figure 9C]. The identified TFs, miRNAs, and chemicals are listed in Supplementary Table 4.
Figure 9. Construction of regulatory networks for prognostic PRGs. (A) mRNA-TF regulatory network. (B) mRNA-chemical regulatory network. (C) mRNA-miRNA regulatory network. Red circles represent the mRNAs of prognostic PRGs, green hexagons indicate TFs, and blue rectangles represent chemicals, and purple diamonds denote miRNAs. PRGs: Pyroptosis-related genes; TF: transcription factor.
DISCUSSION
Pyroptosis is dual-edged for tumor development. On one hand, it releases cytokines, inducing normal cells to undergo malignant transformation. On the other hand, excessive activation of pyroptosis can promote tumor cell death, making it a potential prognostic and therapeutic target for cancers such as HCC[16]. To better understand its clinical relevance, researchers are developing pyroptosis-related prognostic and diagnostic models[17]. Certain PRG signatures have been found to predict the prognosis of HCC patients[18-20]. However, the precise role of pyroptosis in HCC remains unclear.
In this study, we identified 37 significantly differentially expressed PRGs in HCC and discovered that GSDMD, CASP4, and PYCARD were key nodes in the PPI network. Mutation analysis revealed that DHX9, NLRP3, and NLRP7 had the highest mutation frequencies. Notably, a previous study also reported that DHX9 exhibited high expression and frequent amplification in HCC and was involved in DNA damage repair[21]. Our qRT-PCR results showed that 9 PRGs had elevated expression levels in paracancerous tissues compared to normal liver tissues. The relative expression of CASP6, NOD2, and CASP9 in liver cancer tissues was significantly lower than in paracancerous or normal liver tissues. Since pyroptosis can release immune factors and stimulate normal cells to transform into tumor cells, PRGs highly expressed in paracancerous tissue may function in the initiation or development of HCC.
Based on the expression profiles of 49 PRGs, we divided TCGA-HCC samples into two subtypes (C1 and C2). Subtype C2 had more grade III-IV patients and higher immune scores, indicating a worse prognosis. Additionally, subtype C2 showed greater sensitivity to Axitinib and Erlotinib compared to subtype C1. Axitinib is a VEGFR inhibitor, and Erlotinib is an EGFR inhibitor - both have shown therapeutic efficacy in HCC[22,23]. Erlotinib can induce apoptosis and autophagy in HCC cells with various EGFR mutations[24], and EGFR activation has been shown to trigger the NLRP3 pathway, thereby promoting pyroptosis[25]. Therefore, the greater efficacy of Erlotinib in subtype C2 may be related to its sensitivity to EGFR mutations, which influence the pyroptosis pathway.
To enhance clinical applicability, we constructed a prognostic risk model based on four prognostic PRGs (TREM2, GZMA, CNOT6, and NOD2) via LASSO Cox regression analysis. TREM2 acts as an immunomodulatory receptor in infectious diseases by inhibiting caspase-1-dependent pyroptosis and NLRP3 inflammasome-mediated macrophage pyroptosis[26,27]. Its upregulation in human HCC tissues suggests a protective role in hepatocarcinogenesis, as TREM2 deficiency leads to more numerous and larger tumors in fibrosis-associated HCC models[28]. Therefore, its protective effect in HCC development may stem from its inhibitory effect on pyroptosis. GZMA mediates pyroptosis and is exclusively expressed in cytotoxic T lymphocytes (CTL) and natural killer (NK) cells[29]. It regulates T cell activity, stimulates IL-6 production in macrophages, activates pSTAT3 in colon cancer cells, and impairs the efficacy of PD-1 antibody therapy in HCC by disrupting GZMA-F2R interaction, thereby blocking JAK2/STAT1 signaling[30,31]. CNOT6, a transcriptional regulator, has been identified as a key RNA-binding protein related to HCC prognosis, making it a promising prognostic biomarker and therapeutic target[32,33]. NOD2, an innate immune sensor, initiates robust immune responses against pathogens. In HCC, its expression is either lost or significantly downregulated. NOD2 inhibits tumor progression by activating the AMPK pathway, which induces apoptosis[34]. However, the interactions among these four prognosis-related genes in the context of pyroptosis during HCC development remain to be further investigated.
Another important finding of our study is that patients in the high-risk group had a higher proportion of macrophages. Macrophages are abundantly infiltrated in the HCC microenvironment and regulate malignant growth by modulating immune responses and secreting cytokines[35]. Our correlation analysis revealed a positive association between prognostic PRGs and M1 macrophages, and a negative correlation with M2 macrophages. Additionally, M0 Macrophages showed a significant negative correlation with GZMA expression. These findings suggest a potential role for PRGs in regulating macrophage polarization. Furthermore, the observed correlations between prognostic PRGs and macrophage pyroptosis in HCC highlight the impact of immune cells in tumor progression. TREM2-positive tumor-associated macrophages were found to be highly enriched in HCC tumors, rather than in adjacent liver tissue, indicating an immunosuppressive role for TREM2 in the liver tumor microenvironment. The presence of TREM2-positive macrophages expressing FOLR2, CD163, and C1Q-C, along with genes associated with a lipid-associated macrophage-like signature, has been linked to shorter survival in HCC patients[36]. Extracellular GZMA has been shown to induce IL-6 production in M1 macrophages via NF-kB signaling, suggesting that GZMA may indirectly contribute to macrophage pyroptosis[30]. NOD2 positively regulates NLRP3, a key component involved in macrophage pyroptosis[37]. Moreover, TREM2 functions as an immunomodulatory receptor in part through NLRP3 inflammasome-mediated macrophage pyroptosis[31]. Taken together, these findings indicate that patients in the high-risk group, characterized by elevated macrophage infiltration and pronounced macrophage pyroptosis, tend to have shorter survival times.
Finally, we established interaction networks between the mRNAs of prognostic PRGs and corresponding miRNAs, TFs, and chemicals. However, the correlation between PRG expression and drug sensitivity in HCC requires further investigation. Previous studies have indicated that TREM2 knockdown promotes the infiltration and activation of peripheral immune cells and enhances the efficacy of PD-1 blockade in HCC[38]. Additionally, tumor heterogeneity can impair GZMA-F2R signaling, thereby reducing the effectiveness of PD-1 monoclonal antibody therapy through downregulation of the JAK2/STAT1 pathway[31]. Moreover, NOD2 activation has been found to increase the sensitivity of HCC cells to sorafenib, lenvatinib, and 5-FU by activating the AMPK pathway and inducing apoptosis[34]. These findings suggest that targeting prognostic PRGs may enhance the efficacy of both immunotherapy and chemotherapy in HCC.
Despite these promising results, this study has some limitations. We have not directly verified the role of PRGs in HCC, which warrants future exploration. Additionally, we employed unsupervised clustering and LASSO-Cox regression for our analysis. Incorporating more advanced machine learning algorithms for clustering[39,40] could potentially enhance analytical precision and yield deeper insights into underlying data patterns.
In summary, our comprehensive analysis demonstrates the strong association between pyroptosis and HCC. We successfully developed a prognostic risk model for HCC based on four PRGs, which can predict patient outcomes and offer valuable guidance for the development of novel targeted therapies.
DECLARATIONS
Authors’ contributions
Designed and organized the manuscript: Zhou J
Contributed to data acquisition and analysis: Tian L
Wrote the manuscript: He J
Revised the manuscript: Chen W
Investigated the data: Pei Y, Liang Q
All authors read and approved the submitted version.
Availability of data and materials
The data that support the findings of this study are available from the corresponding authors upon reasonable request.
Financial support and sponsorship
This work was supported by grants from The Medical Clinical Key Specialty Construction Project for the 14th Five-Year Plan of Guangdong Province and the Medical High Level - Key Specialty Construction Project for the 14th Five-Year Plan of Foshan City.
Conflicts of interest
All authors declared that there are no conflicts of interest.
Ethics approval and consent to participate
The study was conducted in accordance with the Declaration of Helsinki. The research was reviewed and approved by the Ethics Committee of The First People’s Hospital of Foshan, Lunshenyan (2025) No. 54. All the patients provided written informed consent and patient anonymity has been preserved.
Consent for publication
Not applicable.
Copyright
© The Author(s) 2025.
Supplementary Materials
REFERENCES
1. Gao YX, Yang TW, Yin JM, et al. Progress and prospects of biomarkers in primary liver cancer (review). Int J Oncol. 2020;57:54-66.
3. Balogh J, Victor D 3rd, Asham EH, et al. Hepatocellular carcinoma: a review. J Hepatocell Carcinoma. 2016;3:41-53.
4. Suresh D, Srinivas AN, Kumar DP. Etiology of hepatocellular carcinoma: special focus on fatty liver disease. Front Oncol. 2020;10:601710.
5. Jiang Y, Han QJ, Zhang J. Hepatocellular carcinoma: mechanisms of progression and immunotherapy. World J Gastroenterol. 2019;25:3151-67.
7. Shi J, Zhao Y, Wang K, et al. Cleavage of GSDMD by inflammatory caspases determines pyroptotic cell death. Nature. 2015;526:660-5.
8. Lu F, Lan Z, Xin Z, et al. Emerging insights into molecular mechanisms underlying pyroptosis and functions of inflammasomes in diseases. J Cell Physiol. 2020;235:3207-21.
9. Xia X, Wang X, Cheng Z, et al. The role of pyroptosis in cancer: pro-cancer or pro-"host"? Cell Death Dis. 2019;10:650.
10. Wu Y, Zhang J, Yu S, et al. Cell pyroptosis in health and inflammatory diseases. Cell Death Discov. 2022;8:191.
11. Chu Q, Jiang Y, Zhang W, et al. Pyroptosis is involved in the pathogenesis of human hepatocellular carcinoma. Oncotarget. 2016;7:84658-65.
12. Wei Q, Mu K, Li T, et al. Deregulation of the NLRP3 inflammasome in hepatic parenchymal cells during liver cancer progression. Lab Invest. 2014;94:52-62.
13. Meng J, Huang X, Qiu Y, et al. Pyroptosis-related gene mediated modification patterns and immune cell infiltration landscapes in cutaneous melanoma to aid immunotherapy. Aging. 2021;13:24379-401.
14. Xiang R, Ge Y, Song W, Ren J, Kong C, Fu T. Pyroptosis patterns characterized by distinct tumor microenvironment infiltration landscapes in gastric cancer. Genes. 2021;12:1535.
15. Karki R, Kanneganti TD. Diverging inflammasome signals in tumorigenesis and potential targeting. Nat Rev Cancer. 2019;19:197-214.
16. Wang L, Qin X, Liang J, Ge P. Induction of pyroptosis: a promising strategy for cancer treatment. Front Oncol. 2021;11:635774.
17. Rao Z, Zhu Y, Yang P, et al. Pyroptosis in inflammatory diseases and cancer. Theranostics. 2022;12:4310-29.
18. Zhang S, Li X, Zhang X, Zhang S, Tang C, Kuang W. The pyroptosis-related gene signature predicts the prognosis of hepatocellular carcinoma. Front Mol Biosci. 2021;8:781427.
19. Fu XW, Song CQ. Identification and validation of pyroptosis-related gene signature to predict prognosis and reveal immune infiltration in hepatocellular carcinoma. Front Cell Dev Biol. 2021;9:748039.
20. Liu S, Shao R, Bu X, Xu Y, Shi M. Identification of the pyroptosis-related gene signature for overall survival prediction in patients with hepatocellular carcinoma. Front Cell Dev Biol. 2021;9:742994.
21. Chen X, Lin L, Chen G, et al. High levels of DEAH-box helicases relate to poor prognosis and reduction of DHX9 improves radiosensitivity of hepatocellular carcinoma. Front Oncol. 2022;12:900671.
22. Zhang J, Zong Y, Xu GZ, Xing K. Erlotinib for advanced hepatocellular carcinoma. A systematic review of phase II/III clinical trials. Saudi Med J. 2016;37:1184-90.
23. Kang YK, Yau T, Park JW, et al. Randomized phase II study of axitinib versus placebo plus best supportive care in second-line treatment of advanced hepatocellular carcinoma. Ann Oncol. 2015;26:2457-63.
24. Sueangoen N, Tantiwetrueangdet A, Panvichian R. HCC-derived EGFR mutants are functioning, EGF-dependent, and erlotinib-resistant. Cell Biosci. 2020;10:41.
25. Tan Y, Chen Q, Li X, et al. Pyroptosis: a new paradigm of cell death for fighting against cancer. J Exp Clin Cancer Res. 2021;40:153.
26. Qu W, Wang Y, Wu Y, et al. Triggering receptors expressed on myeloid cells 2 promotes corneal resistance against pseudomonas aeruginosa by inhibiting caspase-1-dependent pyroptosis. Front Immunol. 2018;9:1121.
27. Wang Y, Cao C, Zhu Y, et al. TREM2/β-catenin attenuates NLRP3 inflammasome-mediated macrophage pyroptosis to promote bacterial clearance of pyogenic bacteria. Cell Death Dis. 2022;13:771.
28. Esparza-Baquer A, Labiano I, Sharif O, et al. TREM-2 defends the liver against hepatocellular carcinoma through multifactorial protective mechanisms. Gut. 2021;70:1345-61.
29. Schanoski AS, Le TT, Kaiserman D, et al. Granzyme A in chikungunya and other arboviral infections. Front Immunol. 2020;10:3083.
30. Santiago L, Castro M, Sanz-Pamplona R, et al. Extracellular granzyme a promotes colorectal cancer development by enhancing gut inflammation. Cell Rep. 2020;32:107847.
31. Gao Y, Xu Q, Li X, et al. Heterogeneity induced GZMA-F2R communication inefficient impairs antitumor immunotherapy of PD-1 mAb through JAK2/STAT1 signal suppression in hepatocellular carcinoma. Cell Death Dis. 2022;13:213.
32. Huang Y, Chen S, Qin W, et al. A novel RNA binding protein-related prognostic signature for hepatocellular carcinoma. Front Oncol. 2020;10:580513.
33. Zhao H, Chen C, Chen X, et al. Analysis of CNOT family gene expression, clinicopathological features, and prognosis value in hepatocellular carcinoma. DNA Cell Biol. 2020;39:5818.
34. Ma X, Qiu Y, Sun Y, et al. NOD2 inhibits tumorigenesis and increases chemosensitivity of hepatocellular carcinoma by targeting AMPK pathway. Cell Death Dis. 2020;11:174.
35. Tian Z, Hou X, Liu W, Han Z, Wei L. Macrophages and hepatocellular carcinoma. Cell Biosci. 2019;9:79.
36. Zhou L, Wang M, Guo H, et al. Integrated analysis highlights the immunosuppressive role of TREM2+ macrophages in hepatocellular carcinoma. Front Immunol. 2022;13:848367.
37. Shi CX, Wang Y, Chen Q, Jiao FZ, Pei MH, Gong ZJ. Extracellular histone H3 induces pyroptosis during sepsis and may act through NOD2 and VSIG4/NLRP3 pathways. Front Cell Infect Microbiol. 2020;10:196.
38. Wang Q, Zheng K, Tan D, Liang G. TREM2 knockdown improves the therapeutic effect of PD-1 blockade in hepatocellular carcinoma. Biochem Biophys Res Commun. 2022;636:140-6.
39. Raza A, Bardhan S, Xu L, et al. A machine learning approach for predicting defluorination of per- and polyfluoroalkyl substances (PFAS) for their efficient treatment and removal. Environ Sci Technol Lett. 2019;6:624-9.
Cite This Article

How to Cite
Download Citation
Export Citation File:
Type of Import
Tips on Downloading Citation
Citation Manager File Format
Type of Import
Direct Import: When the Direct Import option is selected (the default state), a dialogue box will give you the option to Save or Open the downloaded citation data. Choosing Open will either launch your citation manager or give you a choice of applications with which to use the metadata. The Save option saves the file locally for later use.
Indirect Import: When the Indirect Import option is selected, the metadata is displayed and may be copied and pasted as needed.
About This Article
Copyright
Data & Comments
Data
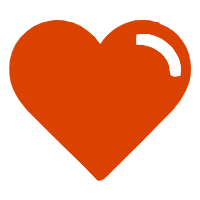
Comments
Comments must be written in English. Spam, offensive content, impersonation, and private information will not be permitted. If any comment is reported and identified as inappropriate content by OAE staff, the comment will be removed without notice. If you have any queries or need any help, please contact us at [email protected].