Unveiling the mechanisms and implications: how artificial intelligence drives green growth in China’s Huaihe River Ecological Economic Belt under the carbon neutrality agenda
Abstract
Amidst the backdrop of global climate warming and China’s proactive chase of its carbon peak and carbon neutrality goals, the Huaihe River Basin (HRB), a region of significant strategic importance in the heartland and eastern expanse of the nation is confronted with formidable challenges, including high energy consumption and severe environmental pollution. Despite its substantial contributions to economic development, the traditional development model of the HRB conflicts with the principles of green development, necessitating the urgent exploration of innovative pathways to sustainable progress. Through a comprehensive review of scholarly literature and rigorous theoretical analysis, this study demonstrates that artificial intelligence (AI) can significantly drive green development by enhancing eco-innovation and optimizing industrial structures. Using a panel dataset from 27 cities in the Huaihe River Ecological Economic Belt (HEB) from 2010 to 2022, this study employs a bidirectional fixed-effects model to analyze the repercussions of AI on green development. The baseline regression results show that for every one-unit increase in AI development level (AIDL), HEB’s urban green development level significantly increases by 0.087. This positive influence is further confirmed through robustness tests. We found that AI can indirectly influence the mechanism and pathway of green development through intermediate variables. AI drives green development indirectly through two pathways: green technology innovation and the rationalization of the industrial structure, with a total explanatory power of 56.7% (R2 = 0.812). Based on these findings, we propose vigorously promoting the green effects of AI, refining industrial structures, and leveraging mediating effects to foster sustainable regional development. These insights offer novel perspectives for the green development of the HRB but also provide valuable references for the green transformation of other areas with similar challenges.
Keywords
INTRODUCTION
Artificial Intelligence (AI) has demonstrated formidable potential in catalyzing global green development as a vanguard technology in the contemporary scientific realm, thereby emerging as a focal point of academic inquiry[1-3]. On the one hand, AI can optimize the energy management system by analyzing energy-related data to predict energy demand and enhance utilization efficiency. It can also improve the accuracy of environmental monitoring, identifying pollution sources and biodiversity changes through sensor and satellite data. Moreover, AI facilitates traditional industries’ green transformation, promotes emerging green industries’ development, and helps predict climate change trends and formulate corresponding strategies. AI also holds substantial promise for facilitating green transformation. Through intelligent algorithms, AI can significantly enhance waste recycling efficiency, fostering the establishment of a closed-loop economic system. In transportation and construction, AI-enabled intelligent control systems optimize energy use, effectively reducing consumption. Moreover, leveraging its high computational power, AI conducts sophisticated climate simulations, providing robust scientific support for decision-making in low-carbon development and ecological conservation. These capabilities collectively position AI as a pivotal driver for sustainable economic and social development. It has been proven to be a catalyst for high-quality economic development and a booster of regional economic quality[4]. Conversely, AI’s high energy consumption in the training phase contrasts with its green aspirations[5], fueling skepticism. Meanwhile, the “rebound effect”[6] during its application may reduce the effectiveness of green development. Against this backdrop, delving into the green development effects of AI in specific regions and uncovering its underlying mechanisms emerges as both crucial and urgent.
As the world’s largest developing country, China has shown strong commitment and proactive measures in addressing global climate change. From setting the goals of achieving carbon peak by 2030 and carbon neutrality by 2060[7], to integrating these ambitions into the overarching framework of ecological civilization, China is actively charting a green and low-carbon development path that aligns with its national conditions. Regional economies’ green transformation and development have emerged as a crucial link in this process. As the cradle of human civilization and a witness to the evolution of the economic division of labor, river basins are vital components of regional economic systems. They serve as key supports for
However, the Huaihe River Ecological Economic Belt (HEB), a crucial nexus spanning the Yangtze and Yellow River Basins, is pivotal to achieving high-quality development in China’s central and eastern regions. Officially endorsed by the State Council as of 2018, this initiative aims to facilitate the ascent of the central region plains economic region and establish itself as China’s fourth central economic growth pole, following the Pearl River Delta, the Yangtze River Delta, and the Bohai Rim[9]. On the one hand, the Huaihe River Basin (HRB) occupies a strategically significant position, making substantial contributions to national economic growth; on the other hand, the traditional development paradigm has subjected the HEB to numerous challenges, including high energy consumption, environmental pollution, and ecological degradation. The prevalence of energy-intensive industries, low resource utilization efficiency, and frequent environmental issues have contradicted the contemporary philosophy of green development. Therefore, there is an urgent need to identify an innovative pathway to break through the current predicament.
In response to this pressing reality, the present study focuses on the HEB and probes the role of AI in propelling green development within this region. Compared to other river basins, theoretical and empirical research on this topic in the HRB remains relatively limited. Building on existing studies, this research strategically targets the underexplored intersection of green development and AI within the HRB, filling critical knowledge gaps in the current academic discourse and exploring the pivotal role of AI in advancing green development in this region. A meticulous exploration of the underlying connection between AI and the green development of the HEB can offer valuable reference cases for other similar areas and even the entire country in addressing the challenge of carbon neutrality and achieving green transformation.
The structure of this paper is as follows: Section 2 offers a concise yet comprehensive review of the existing literature concerning the intersection of AI and the HRB. Section 3 delves into the intricate mechanisms underlying AI, elucidating its operational dynamics and potential impacts. Section 4 outlines the methodologies employed and the data meticulously curated to underpin our analysis. Section 5 presents the empirical findings, complemented by a thorough dissection of the underlying mechanisms. Lastly, Section 6 engages in a profound discussion of the implications of our results for policy formulation and future research endeavors.
LITERATURE REVIEW
The swift expansion of the traditional economy within the HRB not only catalyzes regional development but also engenders severe environmental pollution issues, rendering it one of China’s most contaminated river basins[10]. As the nation increasingly prioritizes ecological and environmental protection, in October 2018, the State Council approved the Development Plan for the HEB, thereby elevating it to the status of a national strategy. This pivotal decision has garnered widespread attention and ignited a surge of academic interest in the high-quality development of the HEB[11]. After 70 years of governance, the HRB has developed a basin governance and water resource utilization system adapted to a well-off society. Cheng et al. confirmed that ecological protection and green development are rising[12]. In this context, outstanding scholars in the academic field have conducted meticulous and in-depth explorations of the paths of green development. They believe that water pollution prevention and control capacity is closely related to water resource utilization efficiency. Wang et al. found that it is also closely linked to the productivity of enterprises[13], and Jalil et al. discovered that it could also have a positive impact on environmental quality through the optimization of the industrial structure[14]. Alexander found that local institutional differences play an essential role in the mechanism by which natural resources affect economic growth and, together with national environmental policies and governance mechanisms, influence environmental quality[15]. Therefore, Ma et al., through empirical testing, argued that to promote coordinated development in the HEB, differentiated strategies should be implemented, investment in environmental governance should be increased, and industrial upgrading and factor optimization should be advanced[16]. The regional development orientation, driving forces, and spatial patterns should be redefined to achieve positive interactions between environmental health and economic vitality. The above research indicates that the HEB focuses on its green development strategy.
The essence of the green development philosophy is rooted in a people-centered approach, emphasizing resource conservation and environmental protection. It advocates for the harmonious and environmentally sound advancement of the socio-economic fabric, human collectivity, and ecology while highlighting the necessity of balancing economic growth with environmental preservation[17]. As a result, numerous scholars have engaged in comprehensive research from economic[18], social[19], and environmental[20] perspectives to substantiate the positive influence of AI on green development. However, the academic community has not yet reached a consensus on the mechanisms by which AI influences green development.
From an economic perspective, AI drives green development by enhancing total factor productivity. Proponents argue that AI improves total factor productivity by optimizing energy-intensive production processes[21], catalyzing green innovation[22], and transforming employment structures[23]. These mechanisms contribute to increased resource utilization efficiency and technological innovation, thereby advancing the objectives of green development. From an environmental perspective, AI helps to reduce environmental pollution and thus promotes green transformation by influencing the energy composition and improving energy efficiency. Securing an eco-friendly future in the face of climate change hinges on the notable ramifications of green energy on carbon emissions and the ecological footprint[24]. Li et al. believe artificial AI is vital in strengthening urban energy conservation and optimizing the energy structure[25]. Subsequent studies have affirmed this view. Yin et al. have confirmed that AI can effectively reduce energy consumption costs[26]; Zhou et al. have confirmed that it can improve energy efficiency[27]; Li et al. have confirmed that AI can also significantly reduce carbon emission intensity[28]. Lin et al. empirical study on Chinese agricultural enterprises elucidated the underlying mechanisms of AI-driven green development. AI positively impacts corporate environmental performance by spurring green product and process innovation, with green corporate culture amplifying this effect[29]. Wang et al. revealed that AI promotes green development through multiple channels. Directly, it correlates significantly with green innovation, facilitating development. Indirectly, it drives green development by enabling industrial structure upgrading and human capital enhancement. Additionally, AI leverages spatial spillover effects to stimulate green innovation in neighboring regions, thereby accelerating the overall advancement of green development[30].
Through these mechanisms, AI fosters a synergistic interplay between economic progress and environmental stewardship. From a regional perspective, apart from these two channels, AI further promotes green development through spatial spillover effects. Chen et al. found that the development of AI has a radiation-driven impact on the green development performance of spatially related areas[31]. This has been evidenced at the urban level across the nation. Yigitcanlar et al. proved that AI promotes the fair distribution of resources among regional cities[32]. Lin et al. found that AI can improve collaborative innovation efficiency[33], further bolstering regional economies’ sustainability.
At the industry level, Li et al. have also confirmed that AI positively impacts green development[34]. AI is also an impetus behind green innovation[35]and green growth[36]. Despite these advancements, AI is not without its challenges. Luan et al. found that training AI leads to escalating energy consumption[37], and the so-called “rebound effect “was discovered by Lange et al.[6]. Given the multi-faceted and complex nature of these issues, conducting more in-depth exploration and rigorous analysis of them is urgent. The academic community, notably, has a crucial role in fostering a comprehensive understanding of these challenges through sustained dialogue and collaborative inquiry.
In the existing literature, there are still ensuing inadequacies in the research on the repercussions of AI on the green development of the ecological regional economic hub: (1) The examination of the precise mechanisms through which AI exerts its influence on the HEB remains a relatively uncharted territory within the current academic landscape. Despite the growing prominence of AI in various domains, its intersection with the principles and practices of the HEB has yet to be fully explored; (2) The purported promotional effect of AI on green development remains a subject of considerable controversy within the academic and policy communities. While some studies highlight AI’s potential to catalyze economic efficiency and innovation, fostering sustainable growth, others caution that its impact may not be uniformly positive; (3) The predominant focus of extant research on urban development and economic analysis has been skewed toward national and provincial scales, with a notable deficiency in data concerning the HEB within the regional context. The lack of detailed city-level data for the Huaihe Economic Belt significantly hampers the precision of policy-making and the ability to capture the region’s complex economic and environmental dynamics; (4) The predominant approach in existing studies of using automated machines as a proxy for measuring the sophistication of AI is inherently flawed, as it may introduce significant and variable measurement errors. This issue arises because automated machines often serve as a simplified representation of AI capabilities, neglecting AI systems’ complex and multi-faceted nature.
THEORETICAL ANALYSIS
In the digital era, AI is the vanguard of a new upsurge of technological upheaval and industrial metamorphosis[38]. By catalyzing green technology innovation and refining the allocation of industrial structure, AI has drastically enhanced the efficiency of urban green development. This transformative impact is further amplified through its salutary influence on the sustainable development of ecological economic belts. While AI-driven green development holds transformative potential, it is accompanied by critical challenges. Proprietary AI systems risk hindering global collaboration through data monopolization and limited transparency, whereas open-source AI faces funding constraints, intellectual property vulnerabilities, and misuse risks. Furthermore, algorithmic bias may exacerbate spatial inequities by disproportionately directing resources to urban centers, amplifying the urban-rural divide. Concurrently, automation in traditional industries could destabilize employment structures, particularly in regions lacking green job retraining programs. This study investigates these dualities through rigorous modeling to unravel the mechanisms driving AI’s impact on sustainable transitions, with a focus on ethical governance and equitable outcomes. Specifically, AI’s ability to optimize resource utilization, drive innovation in green technologies, and enhance the precision of urban environmental management are critical factors contributing to its positive impact.
Green innovation effect
Green innovation develops slowly due to the problem of high initial investment and lengthy payback duration. Still, the development of AI provides a breakthrough, accelerating technological breakthroughs by improving an innovation-friendly environment and technology externalities and gradually becoming the core driving force to promote environmental governance and green development[39]. First is the enabling role of AI technology. Chen et al. find that AI can empower green innovation, and this enabling effect shows nonlinear characteristics of decreasing empowerment range after a marginal increase to a certain extent[31]. The opposition between economic expansion and environmental preservation is alleviated by optimizing resource allocation and use and strengthening the environment’s fine management. In the meantime, new solutions are provided to mitigate problems involving dynamic environmental pollution surveillance, and the government’s intelligent management ability is enhanced. Bera et al. found through empirical research that cutting-edge technologies such as smart grids and energy-saving technologies can also be used to reduce production energy consumption and refine energy utilization[40]. Open-source green innovation initiatives frequently lack viable commercial frameworks, struggling to secure sustained R&D investment. This financial instability results in suboptimal algorithm refinement for critical systems
Structural adjustment effect
Within the sphere of AI, the strategic optimization of the economic structure not only holds the potential to unlock structural dividends but also serves as a powerful catalyst for the swift and transformative shift of the economy toward a green and carbon-efficient trajectory. By leveraging the advanced capabilities of AI, we can meticulously analyze complex economic patterns, identify inefficiencies, and implement targeted interventions that drive sustainable growth. This intelligent reconfiguration of resources promises to revolutionize traditional industries, fostering a harmonious integration of technological innovation and ecological stewardship. Ultimately, it paves the way for a symbiotic future where economic prosperity and environmental sustainability coexist, heralding a new era of responsible development. First, AI can optimize resource allocation. AI can substantially uplift traditional industries’ production efficiency and market competitiveness by enhancing the intelligence level of conventional industries. Wang et al. demonstrated through empirical evidence that AI can promote the sophisticated calibration of the occupational structure of medium-skilled labor and highly skilled labor, and there is a nonlinear relationship with the employment structure of labor[42]. However, the impact of AI on the labor market is twofold. Bonfiglioli et al. found that, to a certain extent, by replacing part of the low-end labor force, the labor force is promoted to develop in the direction of more interpersonal interaction, complex task processing, and flexible adaptation to optimize resource allocation[23]. If workers who have been replaced cannot transition, they may fall into technological unemployment. Therefore, we should consider this aspect when exploring the mechanisms. Second, AI can promote industrial upgrading. With the traits of deep penetration, data-oriented, and intelligent architecture integration, Li et al. have demonstrated that AI not only encourages the technological novelty of traditional commercial sectors but also promotes the growth of human capital, thus improving the industrial structure and fundamentally enhancing the optimization of China’s industrial structure[25]. In summary, Figure 1 illustrates how the development of AI impacts the green development of the HEB through technology-enabling and technology spillover, resource allocation optimization, and industrial structure upgrading.
MATERIALS AND METHODS
Modeling
A baseline regression model was first established to assess AI’s ramifications on HEB’s green development. Before establishing the model, Stata 16.0 software was used to judge and analyze the panel data preliminarily. The panel data in this paper were tested as balanced panel data, and the Hausman test results showed that the fixed effect model was adopted. To provide additional substantiation for the model’s applicability, the joint significance test of all year dummy variables is undertaken, and the data underscore that the model has both time and regional effects. Given the inherent individual heterogeneity and temporal trends in panel data, the two-way fixed effects model controls individual and time-fixed effects simultaneously. This approach effectively mitigates the influence of time-invariant urban characteristics and individual-invariant temporal shocks, enabling precise identification of the causal relationship between AI and green development. The Hausman test rejects the random effects model. In contrast, the joint significance test confirms significant temporal effects, thus justifying the adoption of the two-way over the one-way fixed effects model. Drawing from the preceding analysis, the bidirectional fixed-effect model is selected to control time and prefecture-level city variables to effectively eliminate the potential repercussions of heteroscedasticity on the results derived from the model. Despite controlling for various confounders, unobservable omitted variables may introduce bias. Future research could use instrumental variables or natural experiments to address endogeneity. The dual fixed effect model is set as follows:
In formula (1), GEDIit represents the green development stratum of the city i within the temporal framework of year t; AIit represents the AI composite development profile of city i in the temporal context of year t; ∑Controlit represents a repertoire of control variables, including per capita GDP, industrial structure, scientific and technological level, opening-up degree, and urbanization rate.
Variable selection
Explained variables
Urban green development level of HEB (GEDI). From the actual development status of 27 cities in the HEB, since the typical resource allocation model permits the principle of efficiency maximization, this decentralized and flexible weight will cause some decision-making units (DMUs) to ignore specific inputs or outputs to attain maximum efficiency, which will lead to unfair resource distribution. Moreover, the traditional data envelopment analysis (DEA) model may lead to inconsistent conclusions due to different weight choices when evaluating different DMUs. Therefore, the traditional DEA model cannot fully show the differences and comparability of green development in cities, while the common set of weights DEA (CSW-DEA) model can solve this problem by a unified weight set.
Therefore, this study refers to the general weight calculation method proposed by Feng et al., namely the CSW-DEA model, to measure the index of the GEDI[43]. CSW-DEA model is a new DEA model based on the principle of non-egoism. This model has a weight set (CSW) for fixed cost allocation. Common weights are derived from the collective input and output of all DMUs and applied across them. Twenty-seven prefecture-level resource-based cities are selected as the research sample, and each prefecture-level city serves as a DMU with consistent input and output types. The K DMU consumes m different inputs
Where ek is the efficiency score of DMUk,
Assuming that (
Thus, the green development level of the 27 cities in the HEB can be precisely calculated. As visualized in Figure 2, the data from 2022 are taken as an example to analyze the divergence in the green development levels of all provinces in the HEB. Notably, the region is confronted with the dual challenges of a relatively backward level of high-quality development and pronounced regional imbalances in green development. This underscores the necessity for a deeper and more intricate comprehension of the foundational dynamics and the development of targeted strategies to address these disparities.
Explanatory variables
AIDL: Breaking from traditional variable selections in prior studies, this document adopts the logarithm of the number of AI firms as a metric representing the AI development level, following the approach of Babina et al.[44]. Through the Python crawler technology, the fuzzy matching of keywords such as “intelligence,” “cloud,” “data,” and “Machine Intelligence Learning” is carried out to gather the multi-dimensional data of AI enterprises in each urban region from 2010 to 2022. The logarithm of the extent of AI enterprises in the city that year is used as a diagnostic tool to judge the local AI development level. This paper selects the AIDL in 2010 (the 9th Five-Year Plan), 2015 (the 11th Five-Year Plan), 2018 (proposed by the HEB), and 2022 for visualization processing. As shown in Figure 3, a chart depicting AI development levels over the four years was created to enable a comparative evaluation of the measured levels across these regions for the duration of the study. In light of the overall trend, the spatial distribution shows unbalanced characteristics. Throughout the entire period, Jiangsu Province consistently exhibits a higher level of AI than the other three provinces. Meanwhile, Anhui Province has the lowest development level. It is speculated that this may be closely related to the economic growth level and geographical location.
Control variables
(1) GDP: the increase in per capita consumption power may intensify resource consumption, which is quantified by the logarithm of per capita GDP; (2) Structure: The upgrading of industrial structure can increase the ratio of the service-oriented business, mitigate the carbon emissions of energy-intensive industries, accelerate the uptake of clean energy, and optimize the allocation of resources, which is assessed by the significance of the value-added contribution of the tertiary sector to the GDP; (3) Stem: the level of science and technology indicates the level of investment in promoting the nurturing of sustainable tech advancements, which is quantified by the ratio of scientific and technological fund disbursement to local public finance fund disbursement; (4) Open: the extent of trade liberalization affects technological progress and international cooperation, but may also exacerbate environmental pollution, which is measured by the actual absorption rate of foreign direct investment in GDP; (5) Urb: The urbanization rate serves as a pivotal indicator of the urbanization process, intricately intertwined with critical aspects such as land use, energy consumption, and environmental challenges. It encapsulates the dynamic transformation of urban landscapes and underscores the multi-faceted implications associated with urban expansion, which is measured by the proportion of permanent residents in the total regional population; (6) ER: According to the “Porter Hypothesis”, strict environmental regulation may force enterprises to carry out technological innovation. The comprehensive utilization rate of general industrial solid waste is selected as the proxy variable; (7) Nre: Adding natural resource endowment as a control variable can avoid estimation bias caused by resource dependence. It is measured by the proportion of employees in the extractive industry to the number of employees in the secondary industry.
Data sources and descriptive statistics
In this study, panel data of 27 cities in the HEB from 2010 to 2022 were selected as research samples, mainly from Anhui Statistical Yearbook (ASY), Shandong Statistical Yearbook (SSY), Jiangsu Statistical Yearbook (JSY), and Hubei Statistical Yearbook (HSY), and carbon emission data delineating each prefecture-level city came from China Carbon Accounting Database (CEADs). Green patent data were obtained from the China Patent Publication Network, a small part of the nonexistent data was supplemented by the linear interpolation technique, and the data with large numerical differences were processed by logarithm.
Descriptive statistical analysis was executed to illuminate the salient attributes of the samples as a whole. The findings revealed that the median caliber of ecological maturity in the HEB was 0.569, characterized by a standard deviation of 0.157, indicating that the overall development level was medium and stable. However, the gap extending from the zenith value of 1.000 to the nadir value of 0.213 revealed the regional development imbalance. The average value of AIDL is 4.975, with a deviation range of 1.536, and the degree of dispersion is large, further highlighting the regional development imbalance. Table 1 presents the fundamental statistical indicators of seven variables, including GEDI, AIDL, and GDP. It covers sample size, mean, standard deviation, minimum, and maximum values and is used to show the data distribution of these variables.
Basic statistical measures of variables
Variables | N | Mean | Sd | Min | Max |
GEDI | 351 | 0.569 | 0.157 | 0.213 | 1.000 |
AIDL | 351 | 4.975 | 1.536 | 1.610 | 9.085 |
GDP | 351 | 10.557 | 0.540 | 9.113 | 11.985 |
Structure | 351 | 3.672 | 0.200 | 2.864 | 4.047 |
Stem | 351 | 0.018 | 0.011 | 0.002 | 0.067 |
Open | 351 | 0.021 | 0.015 | 0.002 | 0.078 |
Urb | 351 | 0.510 | 0.103 | 0.291 | 0.739 |
ER | 351 | 0.451 | 0.013 | 0.369 | 0.476 |
Nre | 351 | 0.115 | 0.178 | 0.000 | 0.773 |
GDP is 10.557, indicating that the economic growth is moderate and the variations across regions are significant. The mean value of industrial structure is 3.672, and the transformation of some areas is slow. Stem is low, and the difference is large, related to economic, science, and technology investment. Open fluctuates little, but there are obvious regional differences. The mean value of Urb is 0.510, which is generally high.
RESULTS
Baseline regression results
This study adopted the bidirectional fixed-effect model for regression, and the coefficients between variables were below 0.7. The variance inflation factor (VIF) values corresponding to each variable were below 5 to exclude the possibility of multicollinearity among variables. The results showed that explanatory and control variables did not show significant multicollinearity.
Table 2 shows the baseline regression results with control variables added step by step. When the model is specified with only temporal and regional fixed effects and control variables are excluded, the coefficient of the core explanatory variable on AIDL emerges as significantly positive, achieving statistical significance at the 1% level. This underscores the robust influence of AIDL in the initial model configuration, highlighting its pivotal role in driving the observed outcomes. After gradually introducing control variables, the key coefficient remains positive at the 1% significance level. And passed the significance test, indicating that the AIDL can promote the green development of HEB.
Baseline regression results
(1) | (2) | (3) | (4) | (5) | (6) | (7) | |
AIDL | 0.077*** | 0.073*** | 0.073*** | 0.086*** | 0.087*** | 0.086*** | 0.072*** |
(4.361) | (4.192) | (4.084) | (4.847) | (4.846) | 0.090*** | 0.037 | |
GDP | 0.100*** | 0.097** | 0.087** | 0.089** | (2.612) | (1.038) | |
(2.733) | (2.470) | (2.261) | (2.276) | -0.142*** | -0.133*** | ||
Structure | -0.166*** | -0.172*** | -0.142*** | -0.144*** | (-2.730) | (-2.622) | |
(-3.854) | (-3.328) | (-2.833) | (-2.855) | 0.242 | 0.287 | ||
Stem | 0.211 | 0.289 | 0.273 | (0.384) | (0.466) | ||
(0.278) | (0.391) | (0.368) | -1.835*** | -1.696*** | |||
Open | -1.847*** | -1.843*** | (-3.998) | (-3.783) | |||
(-3.343) | (-3.320) | -0.062 | -0.043 | ||||
Urb | -0.065 | (-0.396) | (-0.281) | ||||
(-0.423) | -0.122 | 0.287 | |||||
ER | (-0.341) | (0.794) | |||||
0.090*** | 0.037 | ||||||
Nre | (2.612) | (1.037) | |||||
-0.141*** | -0.132*** | ||||||
Cons | 0.188** | -0.238 | -0.188 | -0.222 | -0.207 | -0.171 | 0.185 |
(2.159) | (-0.539) | (-0.362) | (-0.443) | (-0.411) | (-0.387) | (0.420) | |
N | 351 | 351 | 351 | 351 | 351 | 351 | 351 |
Fixed time | Yes | Yes | Yes | Yes | Yes | Yes | Yes |
Regional fixation | Yes | Yes | Yes | Yes | Yes | Yes | Yes |
adj. R2 | 0.791 | 0.804 | 0.803 | 0.812 | 0.812 | 0.811 | 0.821 |
Integrating control variables significantly enhances the constructive effect of AI on the GEDI. The negative coefficients of industrial structure, urbanization rate, and openness to the outside world indicate that capital preferentially flows to AI industries. In contrast, excessive urbanization and openness may exacerbate environmental pollution. The positive coefficient of GDP per capita supports the Kuznets curve hypothesis that environmental conditions will improve after a particular stage of economic resurgence. The progress of scientific and technological endeavors has no discernible effect on green development. AI improves resource efficiency, reduces pollution, promotes coordinated regional economic growth, and provides technical support for sustainable development in various ways.
Robustness test
The robustness test is conducted on the baseline regression results to ensure the correctness of the model estimation outcomes. Specific methods are as follows:
(1) Changing the regression model: This paper selects the Tobit model for a robustness test to oversee the sample selection bias caused by the dependent variable being restricted by certain constraints. It can be seen that the use of Tobit regression does not change the soundness of the empirical estimates, and the AIDL will still substantially boost the GEDI.
(2) Winsorization: To mitigate the potential distortion of regression results caused by outliers, the dependent variables in this study are subjected to a 1% Winsorization procedure. The corresponding results are presented in Column (2).
(3) Interactive fixed effects: Considering that there are unobservable factors that fluctuate across various time points at the provincial level, the joint fixed effects of time × province and region × province are further controlled based on the foundational regression model employed as a baseline, and the end products are depicted in Columns (3) and (4).
(4) Lagged explanatory variable: Considering the lagged effect of the development of AI on the GEDI, the AIDL, the principal explanatory variable, is subjected to a one-period temporal displacement.
(5) Sample Time Adjustment: Considering that the core explanatory variables may have measurement bias during the epidemic, the sample time is adjusted, and only the data from 2010 to 2020 are retained for analysis, and the results are shown in Column (6).
(6) Sub-sample regression: four cities of Yancheng, Huai’an, Bengbu, and Xinyang in the sustainable development initiatives nexus of the downstream region of the downstream territories of the Huaihe River are selected to observe the most straightforward effect of AIDL on the GEDI, and the results are shown in Column (7).
Table 3 shows the results of the robustness test. As shown in the table, the robustness test results collectively reaffirm the conclusion that AI functions as a pivotal and foundational element in underpinning the environmental sustainability of the HEB.
Results from the robustness scrutiny
(1) | (2) | (3) | (4) | (5) | (6) | (7) | |
AIDL | 0.087*** | 0.086*** | 0.071*** | 0.087*** | 0.110*** | 0.140** | |
(3.403) | (4.593) | (3.445) | (4.908) | (4.375) | (2.491) | ||
AIDL lagged by one period | 0.062*** | ||||||
(3.548) | |||||||
Cons | 0.126 | -0.341 | -0.366 | -0.207 | 0.138 | -0.206 | 3.169 |
(0.144) | (-0.690) | (-0.754) | (-0.480) | (0.286) | (-0.384) | (1.617) | |
Fixed time | Yes | Yes | Yes | Yes | Yes | Yes | Yes |
Regional fixation | Yes | Yes | Yes | Yes | Yes | Yes | Yes |
Time×Province | Yes | No | |||||
Region×Province | No | Yes | |||||
N | 351 | 344 | 351 | 351 | 324 | 297 | 52 |
adj. R2 | 0.821 | 0.814 | 0.812 | 0.821 | 0.838 | 0.875 |
Test for endogeneity
To ameliorate the potential endogeneity issues, this study draws upon the Two-stage least squares Estimation Method for testing. The mean value of AIDL in other cities in the same province (IV1) and the log value of AIDL enterprise stock (IV2) are selected as instrumental variables. From columns (1) and (3) in the table, it is evident that the two instrumental variables have successfully passed both the weak instrument test and the underidentification test, and both are markedly positive at the level of 1%. Table 4 shows the regression results of the endogeneity test. The regression coefficients in columns (2) and (4) are still significantly positive at 1%. In panel data, traditional instrumental variables (IV) may not effectively deal with the bias caused by the correlation between individual fixed effects and lagged terms. Therefore, after using instrumental variable regression to address the endogeneity issue, the system generalized method of moments (GMM) approach is further employed for endogeneity testing to enhance the robustness and credibility of the estimation. The regression results of the system GMM model show that the first-order lag term of the explanatory variable x and the explained variable y both have a significant positive impact on y, and the model as a whole is significant. The results above reveal that, within the sample period under examination, the salutary influence of AI on the GEDI remains significantly pronounced, even after the potential endogenous biases have been meticulously mitigated through the application of the instrumental variable method. This underscores the robust and enduring positive correlation between AI advancements and the trajectory of green economic development, reinforcing that AI catalyzes sustainable progress in this domain.
Endogeneity test results
Variables | (1) AIDL | (2) GEDI | (3) AIDL | (4) GEDI | (5) GMM |
AIDL | 0.153*** | 0.085*** | 0.013*** | ||
(4.140) | (4.800) | (4.629) | |||
L1.GEDI | 1.126*** | ||||
(22.104) | |||||
The first stage regresses IV1 | 1.753*** | ||||
(9.820) | |||||
The first stage regresses IV2 | 0.970*** | ||||
(121.300) | |||||
Cons | -8.952*** | 0.595*** | |||
(-5.210) | (8.900) | ||||
Controls | Yes | Yes | Yes | Yes | |
Fixed time | Yes | Yes | Yes | Yes | |
Regional fixation | Yes | Yes | Yes | Yes | |
N | 351 | 351 | 351 | 351 | 324 |
Anderson canon. Corr. LM | 84.070*** | 349.760*** | |||
Cragg-Donald Wald F | 96.376 | 86283.510 | |||
Wald statistic | 16.380 | 16.380 | |||
AR(1) | 0.076 | ||||
AR(2) | 0.662 |
Test of the mechanism
Acting as a crucial catalyst for a fresh epoch of scientific and technological disruption and industrial metamorphosis, AI has significantly improved the effectiveness of urban green development and brought about a beneficial transformation in the green development of the ecological, economic enclave through its green technology innovation effect and industrial structure adjustment effect. To investigate the influence of AI on GEDI further, this study uses the mediating effect model to test whether AI affects GEDI by acting on green innovation and structural adjustment. Table 5 shows the baseline regression results of the mechanism test.
Mechanism test results
Variables | Green Innovation | Structural adjustment | ||||||
(1)GPA | (2)GEDI | (3)GPG | (4)GEDI | (5)Ais | (6)GEDI | (7)Thile | (8)GEDI | |
AIDL | -19.769** | 0.090*** | -16.238* | 0.0790*** | 0.013*** | 0.095*** | -0.056** | 0.095*** |
(-2.029) | (4.881) | (-1.716) | (4.420) | (3.874) | (5.034) | (-2.109) | (5.229) | |
GPA | 0.002** | |||||||
(2.055) | ||||||||
GPG | 0.003*** | |||||||
(3.010) | ||||||||
Ais | -0.641* | |||||||
(-1.854) | ||||||||
Thile | 0.144*** | |||||||
(2.963) | ||||||||
Cons | 1,109.687*** | -0.376 | 1,216.637*** | -0.534 | -0.405*** | -0.466 | 6.148*** | -1.094* |
(6.201) | (-0.721) | (7.410) | (-1.164) | (-3.873) | (-0.981) | (10.390) | (-1.952) | |
Controls | Yes | Yes | Yes | Yes | Yes | Yes | Yes | Yes |
Fixed time | Yes | Yes | Yes | Yes | Yes | Yes | Yes | Yes |
Regional fixation | Yes | Yes | Yes | Yes | Yes | Yes | Yes | Yes |
N | 351 | 351 | 351 | 351 | 351 | 351 | 351 | 351 |
adj.R2 | 0.796 | 0.813 | 0.732 | 0.805 | 0.986 | 0.814 | 0.830 | 0.818 |
First, in the quest to explore through empirical testing the green innovation effect of AI, this paper calculates the number of green and low-carbon technology patent applications in each region derived from the “GLTPCS” prepared by the State Intellectual Property Office. It uses the occurrence of green patent applications per 10,000 individuals (GPA) to represent the quality of regional green inventiveness. Drawing on the approach of Du et al., the density of green patent grants per 10,000 population (GPG) was used to characterize the number of regional green innovations to illustrate the complexity of green technology innovation[45]. Columns (1) to (4) represent the green innovation effect, and columns (5) to (8) represent the structural adjustment effect. The outcomes listed in columns (1) and (3) show that AIDL has a decisive and transformative impact on green technology innovation. A significant negative interconnection exists when testing the association between core explanatory variables and intermediary variables. In contrast, when testing explained variables, intermediary variables, and core explanatory variables, there is a significant positive correlation, indicating a U-shaped correlation between AI and green technology innovation. In its nascent stages of development, AI demands substantial amounts of energy, thereby increasing carbon emissions. However, energy utilization efficiency improves as technological advancements unfold, reducing carbon emissions. The experiment conducted by Chen et al. shows that both the direct and spillover effects reveal that only when green technology innovation reaches a certain threshold can it positively influence environmental improvement and carbon emission control[46]. Governments and relevant authorities should take proactive measures to ensure that each city’s green innovation level is positioned on the right side of the “inverted U-shaped” curve, thereby fully leveraging the positive impact of green technology. The results presented in columns (2) and (4) highlight that the coefficient levels for GPA and GPG are 0.002 and 0.003, respectively, implying that the evolution of AI has substantially driven the improvement and increment of green innovation output and promoted the green and eco-friendly and sustainable development of the economic belt through the effect of green innovation.
Secondly, to quantify the structural adjustment effects of AI, two critical variables have been meticulously selected: Ais and Thile. Empirical evidence from columns (5) and (7) reveals that AIDL exerts a profound and transformative impact on structural adjustment. Conversely, the results from columns (6) and (8) indicate that Ais, while significant, fail to reject the null hypothesis at the 10% significance level. In stark contrast, Thile emerges as significantly positive at the 1% significance level, thereby underscoring AI’s burgeoning and progressive role in catalyzing structural upgrading and rationalization adjustment. This suggests that, as a high-tech industry, AI has the potential to recalibrate and optimize the architectural integrity of secondary and tertiary industry frameworks. Ultimately, this recalibration is poised to have a more favorable impact on GDP, paving the way for sustainable and innovative economic growth. Figure 4 shows that AI drives the green development of the Huai River Ecological Economic Belt via green innovation quality, quantity, and industrial structure upgrading & rationalization.
DISCUSSION
Research conclusions
In economic transformation, AI, as a crucial determinant that holds sway over the outcome to promote the formation of a new zenith of optimized efficiency and productivity, has great potential to guide China’s economic expansion force and achieve the vision of enduring growth and environmental stewardship. This paper starts from a new perspective of new quality productivity, and uses the cross-sectional time-series data of 27 cities in the HEB from 2010 to 2022. The empirical test explored the catalytic role and operational framework of v on the green development of HEB. The overarching conclusions are elucidated as follows: First, the advancement in AI has profoundly catalyzed the green development trajectory of the Huaihe region. This assertion maintains its validity even when accounting for potential endogenous biases. Second, AI exhibits a uniform impact across diverse temporal and spatial contexts, thereby underscoring its consistent influence. Third, through the conduits of green innovation and structural adjustment, AI exerts a salutary influence on the green development of the Huaihe region. This demonstrates that AI is a crucial catalyst in propelling green technological advancements and facilitating the transition of industries toward an environmentally sustainable structure. Optimizing the structure of industrial sectors and enhancing inter-sectoral coordination can boost economic efficiency and sustainability, facilitating the transition of industries from traditional high-pollution models to AI-integrated green industries. Although this transformation may cause short-term job losses in traditional sectors, it will generate new employment opportunities in AI-driven fields and green industries. This study elucidates the ramifications of AI on the green development of the Huaihe region, deepens the insight into the complex interaction between AI and green development, and reveals the latent role of AI in promoting sustainable economic transformation. This is achieved in tandem with the impetus derived from environmental protection and sustainable development.
Countermeasures and suggestions
First, vigorously develop the green effect of AI. It is essential to unleash AI’s green potential vigorously across the HEB. Enhancing the maturity of AI applications within diverse regions of the HEB and continuously tapping its potential to drive low-carbon, efficient, and climate-resilient development are critical steps. Integrating AI technology with environmental monitoring and protected area management is a key driver for enhancing environmental governance and promoting green development. Yu et al. used a deep ensemble learning model with global data from 5,446 stations to predict PM2.5 concentrations at
Second, the government should restructure the intelligent manufacturing ecosystem and innovation framework to fortify the industrial structure. The government needs to persistently foster the Scientific and Technological development of v and other eco-friendly technologies to catalyze industrial modernization further and ensure that AI maximizes its effectiveness in advancing green development. Drawing on the success of ThyssenKrupp Steel in Germany, the company utilized AI to optimize blast furnace gas ratios in real-time. Coupled with the EU’s “green steel” tax relief policies, it achieved a 22% reduction in carbon emissions per ton of steel, resulting in an annual emission reduction of 500,000 tons. Initially, it is imperative to catalyze the intelligent transformation of traditional industries expeditiously. By leveraging the advanced capabilities of AI, we can judiciously curtail energy consumption and emissions, propelling the manufacturing sector toward a paradigm characterized by high-end innovation, intelligent operations, and environmental benignity. This transformation is a technological upgrade and a strategic pivot toward sustainable industrial practices. Subsequently, there must be a significant augmentation of financial expenditure allocated to the research and development initiatives of AI-driven green technologies. This includes robust support for related scientific research projects and the encouragement of enterprises to engage actively in green technology innovation. By doing so, we can accelerate the diffusion of green technologies and enhance their market penetration. This involves uplifting existing facilities’ energy-saving and carbon-reduction transformation, guiding the green and low-carbon development of digital technology enterprises, and assisting both upstream and downstream enterprises in enhancing their carbon-reduction capabilities. In conclusion, the paramount imperative lies in prioritizing green and low-carbon digital infrastructure construction. This endeavor is not merely a strategic choice but a fundamental necessity in the contemporary era, where the confluence of technological advancement and environmental sustainability defines the trajectory of global progress. By emphasizing the development of such infrastructure, we can ensure that principles of ecological responsibility and long-term viability underpin our digital future. This approach will foster innovation and economic growth and contribute to the broader goal of mitigating environmental impact, thereby aligning our technological ambitions with the imperatives of a sustainable planet. AI’s green potential is universal across industrial, energy, agricultural, and ecological management domains. Its success relies on the synergy of technology, policy, and localization. Developed regions leverage high-quality data and market mechanisms for rapid technological diffusion, while developing countries must strengthen infrastructure and skill training. Compared with the HEB, international cases highlight the efficacy of government-led models in cross-sectoral resource coordination, yet also emphasize the necessity of flexible policies to enhance social acceptance. A global open-source platform for AI green technologies could foster cross-regional collaboration, standardize solutions, and enable regionally tailored innovation. Through these concerted efforts, we can create a resilient and sustainable digital ecosystem that aligns with global environmental goals.
Thirdly, leveraging the mediating effect as a catalyst for indirect advancement is imperative. With its
Future research endeavors may delve deeper into several pivotal directions. Expanding the scope of research regions and conducting comparative analyses of the green development effects of AI across diverse economic and environmental contexts could significantly enhance the generalizability of research conclusions. Secondly, an extensive scrutiny of the specific application pathways of AI technologies within various industries and their differential impacts on green transitions would provide more targeted policy recommendations. Thirdly, integrating big data and machine learning methodologies to monitor the
DECLARATIONS
Authors’ contributions
Conceptualization, supervision, funding acquisition, writing - original draft preparation, writing - reviewing and editing: Zhao, X.
Software, data curation, visualization, writing - original draft preparation, writing - review and editing: Wang, Z.
Resources, writing - review and editing, investigation: Vasa, L.
Validation, writing - review, and editing: Dincer, H.
Availability of data and materials
The data supporting this study’s findings are available from the corresponding author upon reasonable request.
Financial support and sponsorship
This work was supported by the Social Sciences Planning Youth Project of Anhui Province (Grant No. AHSKQ2022D138).
Conflicts of interest
Zhao, X.; Vasa, L.; and Dincer H. are the Guest Editors of the Special Issue titled “Impact of the Digital Economy on Urban Carbon Emission Reduction Strategies: Insights from AI” in the journal Carbon Footprints and were not involved in any part of the editorial process, including reviewer selection, manuscript handling, or decision-making. The other authors declare that they have no conflicts of interest.
Ethical approval and consent to participate
Not applicable.
Consent for publication
Not applicable.
Copyright
© The Author(s) 2025.
REFERENCES
1. Huang, J.; Gopal, S. Green AI-a multidisciplinary approach to sustainability. Environ. Sci. Ecotechnol. 2025, 24, 100536.
2. Huang, L.; Chin, T.; Papa, A.; Pisano, P. Artificial intelligence augmenting human intelligence for manufacturing firms to create green value: Towards a technology adoption perspective. Technol. Forecast. Soc. Change. 2025, 213, 124013.
3. Zhong, K.; Song, L. Artificial intelligence adoption and corporate green innovation capability. Financ. Res. Lett. 2025, 72, 106480.
4. Li, B.; Jiang, F.; Xia, H.; Pan, J. Under the background of AI application, research on the impact of science and technology innovation and industrial structure upgrading on the sustainable and high-quality development of regional economies. Sustainability 2022, 14, 11331.
5. Jones, N. How to stop data centres from gobbling up the world’s electricity. Nature 2018, 561, 163-6.
6. Lange, S.; Pohl, J.; Santarius, T. Digitalization and energy consumption. Does ICT reduce energy demand? Ecol. Econ. 2020, 176, 106760.
7. Liu, Z.; Deng, Z.; He, G.; et al. Challenges and opportunities for carbon neutrality in China. Nat. Rev. Earth. Environ. 2021, 3, 141-55.
8. Cheng, G.; Li, X.; Zhao, W.; et al. Integrated study of the water-ecosystem-economy in the Heihe River basin. Nat. Sci. Revi. 2014, 1, 413-28.
9. Yang, D.; Zhu, C.; Li, J.; et al. Exploring the supply and demand imbalance of carbon and carbon-related ecosystem services for dualcarbon goal ecological management in the Huaihe River Ecological Economic Belt. Sci. Total. Environ. 2024, 912, 169169.
10. Xu, J.; Jin, G.; Mo, Y.; Tang, H.; Li, L. Assessing anthropogenic impacts on chemical and biochemical oxygen demand in different spatial scales with Bayesian networks. Water 2020, 12, 246.
11. Zhang, C.; Wang, C.; Mao, G.; Wang, M.; Hsu, W. An empirical study on the ecological economy of the Huai River in China. Water 2020, 12, 2162.
12. Cheng, Y.; Zhang, D. Spatial and temporal differentiation trends and attributions of high-quality development in the Huaihe Eco-Economic Belt. J. Resour. Ecol. 2023, 14, 517-32.
13. Wang, C.; Wu, J.; Zhang, B. Environmental regulation, emissions and productivity: evidence from Chinese COD-emitting manufacturers. J. Environ. Econ. Manage. 2018, 92, 54-73.
14. Jalil, A.; Feridun, M. The impact of growth, energy and financial development on the environment in China: a cointegration analysis. Energy. Econ. 2011, 33, 284-91.
15. Libman, A. Natural resources and sub-national economic performance: DOEs sub-national democracy matter? Energy. Econ. 2013, 37, 82-99.
16. Ma, X.; Shahbaz, M.; Song, M. Off-office audit of natural resource assets and water pollution: a quasi-natural experiment in China. J. Enterp. Inf. Manag. 2025, 38, 292-317.
17. Liu, P.; Zhong, F.; Han, N. Efficiency and equity: effect of urban agglomerations’ spatial structure on green development efficiency in China. Sustain. Cities. Soc. 2024, 108, 105504.
19. Makridakis, S. The forthcoming artificial intelligence (AI) revolution: its impact on society and firms. Futures 2017, 90, 46-60.
20. Sarkar, M.; Sarkar, B. How does an industry reduce waste and consumed energy within a multi-stage smart sustainable biofuel production system? J. Clean. Prod. 2020, 262, 121200.
21. Wheeldon, A.; Shafik, R.; Rahman, T.; Lei, J.; Yakovlev, A.; Granmo, O. C. Learning automata based energy-efficient AI hardware design for IoT applications. Phil. Trans. R. Soc. A. 2020, 378, 20190593.
22. Liu, X.; Cifuentes-faura, J.; Yang, X.; Pan, J. The green innovation effect of industrial robot applications: evidence from Chinese manufacturing companies. Technol. Forecast. Social. Change. 2025, 210, 123904.
23. Bonfiglioli, A.; Crinò, R.; Gancia, G.; Papadakis, I. Artificial intelligence and jobs: evidence from US commuting zones. Economic. Policy. 2025, 40, 145-94.
24. Tariq, G.; Sun, H.; Ali, S. Environmental footprint impacts of green energies, green energy finance and green governance in G7 countries. Carbon. Footprints. 2024, 3, 5.
25. Li, X.; Wang, Q.; Tang, Y. The impact of artificial intelligence development on urban energy efficiency-based on the perspective of smart city policy. Sustainability 2024, 16, 3200.
26. Yin, K.; Cai, F.; Huang, C. How does artificial intelligence development affect green technology innovation in China? Environ. Sci. Pollut. Res. Int. 2023, 30, 28066-90.
27. Zhou, W.; Zhuang, Y.; Chen, Y. How does artificial intelligence affect pollutant emissions by improving energy efficiency and developing green technology. Energy. Economics. 2024, 131, 107355.
28. Li, Y.; Zhang, Y.; Pan, A.; Han, M.; Veglianti, E. Carbon emission reduction effects of industrial robot applications: heterogeneity characteristics and influencing mechanisms. Technol. Soc. 2022, 70, 102034.
29. Lin, J.; Zeng, Y.; Wu, S.; Luo, X. How does artificial intelligence affect the environmental performance of organizations? Inf. Manag. 2024, 61, 103924.
30. Wang, Q.; Sun, T.; Li, R. Does artificial intelligence promote green innovation? Energy. Environ. 2025, 36, 1005-37.
31. Chen, M.; Wang, S.; Wang, X. How does artificial intelligence impact green development? Sustainability 2024, 16, 1260.
32. Yigitcanlar, T.; Mehmood, R.; Corchado, J. M. Green artificial intelligence: towards an efficient, sustainable and equitable technology for smart cities and futures. Sustainability 2021, 13, 8952.
33. Lin, S.; Wang, M.; Jing, C.; et al. The influence of AI on the economic growth of different regions in China. Sci. Rep. 2024, 14, 9169.
34. Li, T.; Luo, J.; Liang, K.; Yi, C.; Ma, L. Synergy of patent and open-source-driven sustainable climate governance under green AI: a case study of TinyML. Sustainability 2023, 15, 13779.
35. Zhao, X.; Nakonieczny, J.; Jabeen, F.; Shahzad, U.; Jia, W. Does green innovation induce green total factor productivity? Technol. Forecast. Social. Change. 2022, 185, 122021.
36. Qian, Y.; Liu, J.; Shi, L.; Forrest, J. Y.; Yang, Z. Can artificial intelligence improve green economic growth? Environ. Sci. Pollut. Res. Int. 2023, 30, 16418-37.
37. Luan, F.; Yang, X.; Chen, Y.; Regis, P. J. Industrial robots and air environment: a moderated mediation model of population density and energy consumption. Sustain. Prod. Consum. 2022, 30, 870-88.
38. Vinuesa, R.; Azizpour, H.; Leite, I.; et al. The role of artificial intelligence in achieving the sustainable development goals. Nat. Commun. 2020, 11, 233.
39. Budde, M.; Hilbring, D.; Vogl, J.; Dittmar, D.; Abecker, A. NiMo 4.0 - enabling advanced data analytics with AI for environmental governance in the water domain. At. -. Autom. 2024, 72, 564-78.
40. Bera, M.; Das, S.; Garai, S.; et al. Advancing energy efficiency: innovative technologies and strategic measures for achieving net zero emissions. Carbon. Footprints. 2025, 4, 3.
41. Tang, R. Digital economy drives tourism development-empirical evidence based on the UK. Econ. Res. Ekon. Istraž. 2023, 36, 2003-20.
42. Wang, X.; Chen, M.; Chen, N. How artificial intelligence affects the labour force employment structure from the perspective of industrial structure optimisation. Heliyon 2024, 10, e26686.
43. Feng, Q.; Wu, Z.; Zhou, G. Fixed cost allocation considering the input-output scale based on DEA approach. Comput. Ind. Eng. 2021, 159, 107476.
44. Babina, T.; Fedyk, A.; He, A.; Hodson, J. Artificial intelligence, firm growth, and product innovation. J. Financ. Econ. 2024, 151, 103745.
45. Du, K.; Li, P.; Yan, Z. Do green technology innovations contribute to carbon dioxide emission reduction? Technol. Forecast. Soc. Change. 2019, 146, 297-303.
46. Chen, H.; Yi, J.; Chen, A.; Peng, D.; Yang, J. Green technology innovation and CO2 emission in China: evidence from a spatial-temporal analysis and a nonlinear spatial Durbin model. Energy. Policy. 2023, 172, 113338.
47. Yu, W.; Ye, T.; Zhang, Y.; et al. Global estimates of daily ambient fine particulate matter concentrations and unequal spatiotemporal distribution of population exposure: a machine learning modeling study. Lancet. Planet. Health. 2023, 7, e209-18.
48. Chen, G.; Li, S.; Knibbs, L. D.; et al. A machine learning method to estimate PM2.5 concentrations across China with remote sensing, meteorological and land use information. Sci. Total. Environ. 2018, 636, 52-60.
Cite This Article

How to Cite
Download Citation
Export Citation File:
Type of Import
Tips on Downloading Citation
Citation Manager File Format
Type of Import
Direct Import: When the Direct Import option is selected (the default state), a dialogue box will give you the option to Save or Open the downloaded citation data. Choosing Open will either launch your citation manager or give you a choice of applications with which to use the metadata. The Save option saves the file locally for later use.
Indirect Import: When the Indirect Import option is selected, the metadata is displayed and may be copied and pasted as needed.
About This Article
Special Issue
Copyright
Data & Comments
Data
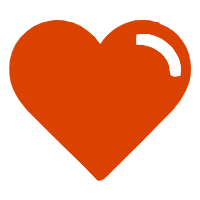
Comments
Comments must be written in English. Spam, offensive content, impersonation, and private information will not be permitted. If any comment is reported and identified as inappropriate content by OAE staff, the comment will be removed without notice. If you have any queries or need any help, please contact us at [email protected].